The Rise of Quantum Robots
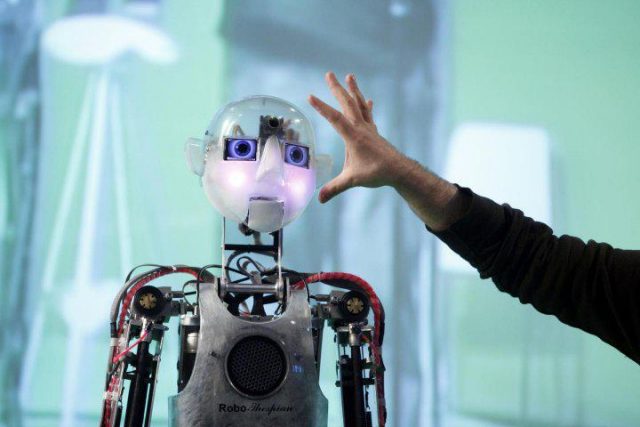
Two of the hot topics in science an technology nowadays are related to Computer Science. They are Artificial Intelligence (AI) and Quantum Computing (QC). AI is a really old field, as humanity has dreamed of having autonomous machines for decades. Quantum Computing is not as old as AI. It is considered that the idea of using quantum systems to compute was first proposed by Richard Feynman in 1982 1. In this post, we will review how the mixing of these two techniques can be world-changing.
In the field of artificial intelligence, several groundbreaking results have been announced recently. One of the critical advances in this field is the capacity of recognising images. This line has led to technologies such as self-driving cars. There are many other recent developments including AlphaGo, Deep Dream, and Hand Talk. Of course, this is not a complete list, and new applications are being developed on a daily basis.
The second revolution we want to discuss is the development of quantum computers. After decades of research, we are in a historic moment because new platforms for quantum computing are being released. It looks like we will have an operating better-than-classical quantum computer soon. This opens the door to new promising applications and technologies.
The mixing of these two fields is quite natural. Artificial intelligence is a current technology with many potential applications, while quantum computers promise a computation power beyond our classical tools. It is evident that the merge of these two technologies may bring us new possibilities. Soon, it would be possible that we have quantum robots performing better than their outdated cousins, the classical robots.
The idea of quantum learning machines dates from several years ago. Then, some toy models were proposed to explore the power of quantum computation in the performance of automatic tasks 23. These tasks were neither complex nor useful. The whole point of the research was just to prove that quantum automata can perform better than classical ones. At this moment of the study, the complexity of the task was not relevant.
More recently, general quantum learning frameworks have been proposed. These proposals are based on already known classical frameworks as reinforcement learning. Hans J. Briegel proposed one of these frameworks in 2012, calling it Projective Simulation 4. His method was based on a random walk on complex graphs, and it can solve well-known AI problems as the grid-world and the mountain-car problem 5.
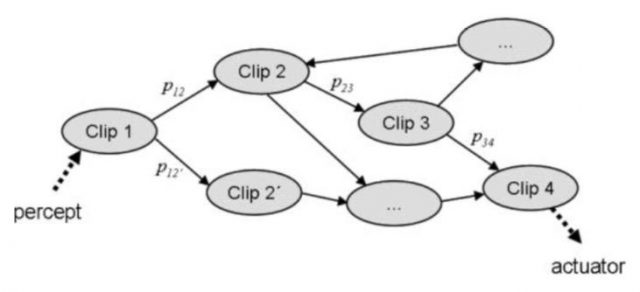
Briegel’s group extended the Projective Simulation to the quantum domain by changing the random walk for a quantum walk. It has been proved that this can make the system quadratically faster than its classical counterpart in the decision time 67. Thinking fast is an advantage for complex problems, as there the internal thinking of the quantum machine can be several orders of magnitude faster than its classical counterpart. In environments that require a rapid response this can give the quantum robots a considerable advantage over their classical cousins.
There are many other AI problems were quantum mechanics can offers an advantage. A comprehensive review can be found in Ref. 8. The following table summarises its results.
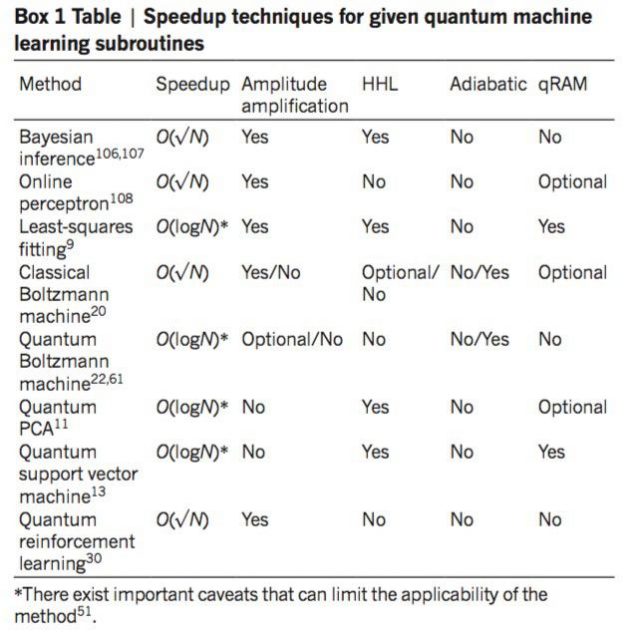
We will discuss only one of the methods mentioned in this review. It is called Quantum Annealing (QA). Although QA is not by itself an AI algorithm, it can play an essential role in the field. This technique is used to minimise function by using the quantum properties of a system. As it is reflected in Figure 2, annealing is based on finding the lowest-energy state of a system. A classical system needs to “jump” over the energy barriers while a quantum system can just tunnel through it. Because of that, quantum annealing is more efficient than the classical one. As minimisation is at the core of most of AI problems this can be a game-changer.
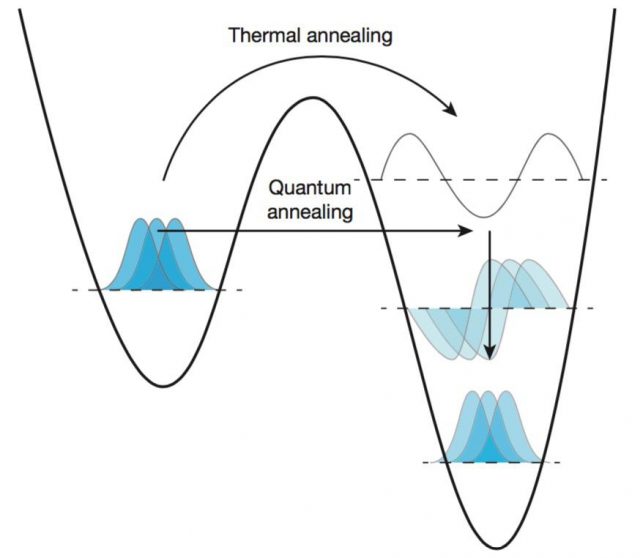
The main importance of this approach resides mainly in the existence of the D-Wave machine. D-Wave performs quantum annealing, and the promises are that it will overpass classical computers shortly. There are still concerns about its capabilities and if it is conducting a genuinely quantum process, but if it were true that in several years D-Wave will perform annealing faster than a classical computer its application in the field of AI will be countless.
These are only some examples, but considering that the field is less than ten years old, it is clear that Quantum AI is one of the hot topics of the moment. Its findings are still only theoretical but in the next years we shall see the rise of quantum robots.
References
- R.P. Feynman. Simulating Physics with Computers. Int. J. Theor. Phys. 21, 467 (1982). ↩
- D. Manzano, M. Pawlowski, C. Brukner. The speed of quantum and classical learning for performing the kth root of NOT. New Journal of Physics 11, 113018 (2009). ↩
- M. Siomau. A quantum model for autonomous learning automata. Quantum Information Processing 13, 1211 (2014). ↩
- H.J. Briegel and G. de las Cuevas. Projective simulation for artificial intelligence. Scientific Reports 2, 400 (2012). ↩
- A.A. Melnikov, A. Makmal, H. J. Briegel. Projective simulation applied to the grid-world and the mountain-car problem. arXiv:1405.5459 (2014). ↩
- G.D. Paparo et al, Quantum Speedup for Active Learning Agents. Phys. Rev. X 4, 031002 (2014). ↩
- V. Djunko, J.M. Taylor, and H.J. Briegel. Quantum-Enhanced Machine Learning. Phys. Rev. Lett. 117, 130501 (2016). ↩
- J. Biamonte, et al. Quantum Machine Learning. Nature 549, 195 (2017). ↩
4 comments
[…] de là à utiliser ces algorithmes dans la robotique comme décrit dans The Rise of Quantum Robots de Daniel Manzano (avril 2018), il faudra patienter un peu ! Ce n’est plus de la technologie, […]
[…] de là à utiliser ces algorithmes dans la robotique comme décrit dans The Rise of Quantum Robots de Daniel Manzano (avril 2018), il faudra patienter un peu ! Ce n’est plus de la technologie, […]
[…] were proposed to explore the power of quantum computation in the performance of automatic tasks 23. These tasks were neither complex nor useful. The whole point of the research was just to prove […]
[…] Hans J. Briegel proposed one of these frameworks in 2012, calling it Projective Simulation 4. His method was based on a random walk on complex graphs, and it can solve well-known AI problems […]