Multiscale mathematical models for glioma progression
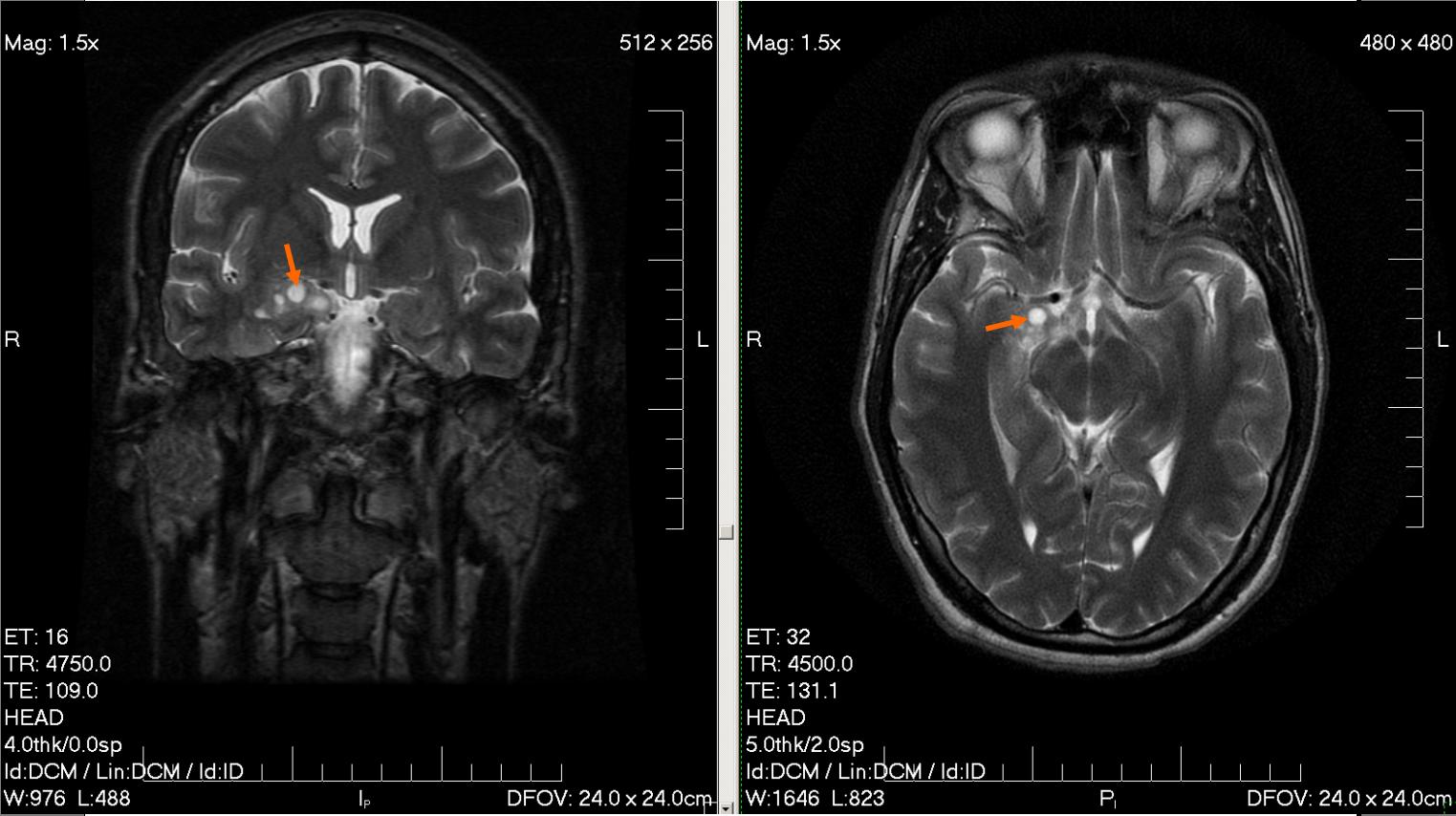
Gliomas are the most prevalent subtype of primary brain tumours originating from mutations of the glia cells in the central nervous system. Gliomas are characterized by fast cell growth, strong invasion capability, and well-developed tumour vasculature. Although research and clinical trials have allowed significant progress in the comprehension and treatment of gliomas, they still have a poor prognosis, with a median survival range between 9 and 12 months. Research on the mechanisms driving glioma progression remains an emerging field.
The use of new technologies has created a considerable amount of clinical and biological data concerning the mechanisms that drive glioma evolution. The need to extract useful information from this data makes mathematical models powerful tools. Exploiting the inherently multiscale nature of glioma evolution allows defining models based on dynamical systems, kinetic equations, and macroscopic partial differential equations with different roles depending on the considered phenomena.
Now, Martina Conte and her collaborators propose 1 different mathematical models for the description of some relevant microscopic and macroscopic mechanisms involved in glioma growth and spread inside the brain, integrating clinical and biological data in the model settings.
The experimental data used were obtained from magnetic resonance and diffusion tensor images of the human brain and from in vivo immunofluorescence analysis of protein distributions in Drosophila, a reliable model for the study of glioblastoma dynamics. The anisotropic characteristics of the brain tissue, using the diffusion tensor data, and the influence of the fibre structures on tumour cell dynamics were analysed and integrated. The researchers show 23 how the fibre network directs cell migration along preferential paths, reproducing the branched and heterogeneous patterns typical of glioma evolution, and how multi-modal treatments can reduce this behaviour.
Conte et al. also study 4 the interdependency of micro-environmental acidity and vasculature in tumour angiogenesis, defining a model capable of reproducing their influence on the emergence of phenotypic heterogeneity and hypoxia-related features (like necrosis) typical of glioma progression.
In a fourth model, the role of cell protrusions from a non-local perspective and their influence on the contact guidance phenomenon and on the emergence of collaborative or competitive effects between two cues driving cell velocity changes was considered 5. The model shows how the biochemical and biomechanical interactions of these agents result in the emergence of tumour propagation fronts, which can evolve in very different ways as a response to environmental changes.
The diverse settings proposed by Conte et al. (micro-meso models, the non-local kinetic model and the macroscopic model) show considerable advances in the glioma modelling field, being able to capture key reported experimental evidence of glioma progression.
Author: César Tomé López is a science writer and the editor of Mapping Ignorance
Disclaimer: Parts of this article might have been copied verbatim or almost verbatim from the referenced research papers.
References
- M. Conte (2021) Mathematical models for glioma growth and migration inside the brain UPV/EHU ↩
- M. Conte, S. Casas-Tinto, and J. Soler. “Modeling invasion patterns in the glioblastoma battlefield”. bioRxiv (2020). DOI: 10.1101/2020.06.17.156497 ↩
- M. Conte, L. Gerardo-Giorda, and M. Groppi. “Glioma invasion and its inter-play with nervous tissue and therapy: A multiscale model”. Journal of Theoretical Biology 486 (2020), p. 110088. DOI: 10.1016/j.jtbi.2019.110088 ↩
- M. Conte and C. Surulescu. “Mathematical modeling of glioma invasion: acid-and vasculature mediated go-or-grow dichotomy and the influence of tissueanisotropy”. arXiv (2020). arXiv:2007.12204v1 ↩
- M. Conte and N. Loy. “Multi-cue kinetic model with non-local sensing for cell migration on a fibres network with chemotaxis”. arXiv (2020). arXiv:2006.09707v2 ↩