Dr. Jekyll and Mr. Hyde: what changes in a stem cell to become malignant?
Author: José R. Pineda got his Ph.D. from University of Barcelona in 2006. Since 2007 he has worked for Institut Curie and The French Alternative Energies and Atomic Energy Commission. Currently he is a researcher of the UPV/EHU. He investigates the role of stem cells in physiologic and pathologic conditions.
Research in oncology advances very fast and currently machine-learning-based expert systems exist for the pathologist to support the diagnosis of tumors of unknown origin 1 or even deep comprehension of the role of both immunotherapy and tumor heterogeneity in immune-tumor interactions 2. However, a key aspect to better understand the problem at its roots is to gain basic knowledge about the changes that overcome in a healthy cell to transform into a cancerous cell. One of the best examples may be the case of stem cells. Stem cells are the paradigm of future cell therapy to regenerate tissues, however cancer-stem like cells are the utmost nightmare very difficult to treat and responsible for aggressive tumor relapses.
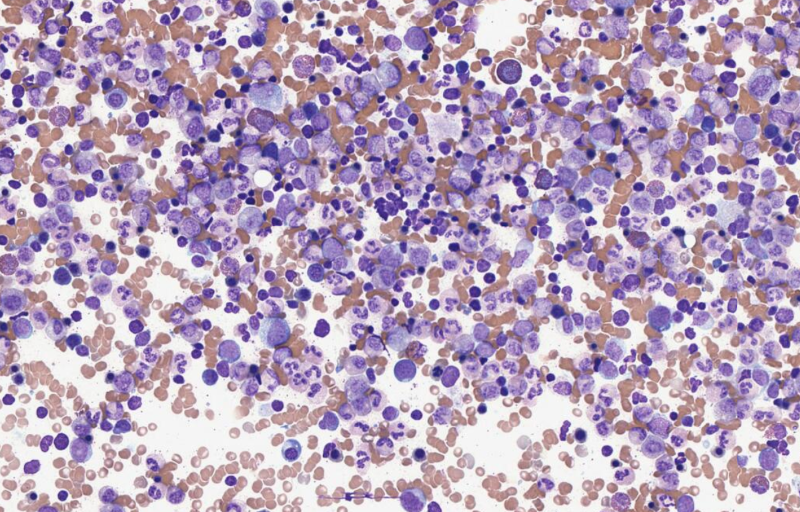
In March 2021 in a collaboration between the Centre for Genomic Regulation (CRG), the European Molecular Biology Laboratory (EMBL) and the Stanford Genome Technology Center, Velten and its collaborators published in the prestigious Nature Communications a multi-omic approach to enable the identification and characterization of cellular changes of hematopoietic stem cells (HSCs) to leukemic stem cells (LSCs) 3. That means, using different disciplines such as genomics, transcriptomic, computational tools and single-cell RNAseq allowed to the researches to distinguish the different steps happening to a healthy cell to transform progressively into a cancerous cell. This proof of concept is equiparabe to “read the thoughts” of a cell and learn new knowledge about the steps required for this transformation.
Velten et al. used the acute myeloid leukemia (AML) model because in hematopoietic stem cells (the cells responsible to generate our blood and immune system) over time and especially during ageing, has been described in these cells the apparition of pre-leukemic mutations 4. That does not mean that all the individuals will develop leukemia, but normal cells carrying these mutations still be able to produce healthy blood and immune cells. However, the increase or acquisition of new mutations could end in an expansion of aberrant stem cells that could lead the initiation of a future leukemia. Cancer stem cells are slowly dividing cells resistant to current treatments and for thus, difficult to target therapeutically, provoking in AML, recurrent tumor relapse with 5-year survival rates below 15% for patients over the age of 60. The side effects of current treatments on normal hematopoietic stem cells are very dangerous, increasing the risk of anemia or infections due to low levels of white blood cells. For all this reasons, the identification of therapeutic strategies that target leukemic stem cells, while sparing healthy hematopoietic stem cells will offer better wellness and lifespan for the patients.
To date, to characterize the patterns of expression of leukemia stem cells specific genes the researchers firstly isolate cells with hematopoietic stem cell-specific surface markers such CD34 and then engraft these cells in xenograft models to correlate with gene expression. However, these approaches measure impure/heterogeneous population of cells over time. To overcome these problems, Velten et al, designed a strategy to characterize the differences on gene expression among three stem cell populations: healthy hematopoietic stem cells (HSCs), pre-leukemia stem cells (pre-LSCs), and leukemia stem cells (LSCs). By measuring mutational status and gene expression in single cells simultaneously, cancer stem cells can be uniquely distinguished from both mature cancer cells (based on gene expression) and healthy stem cells (based on mutational status). Finally, pre-LSCs are assumed to carry mutations not yet associated with fully developed leukemia, enabling their identification by profiling both known leukemic and known pre-leukemic mutations through the detection of clonal hematopoiesis of indeterminate potential (CHIP) mutations.
To identify and amplify “mutational” information for each cell, the researchers used single-cell RNA-seq technique transcribing the complementary DNA (cDNA). In a next step, using MutaSeq (a workflow that amplifies nuclear mutations from cDNA) and mitoClone (a computational tool that achieves high-confidence clonal assignments and de novo discovery of clones using mitochondrial marker mutations when available), the team used genomic and mitochondrial mutations to distinguish between healthy and cancerous cells. The authors used samples from four AML patients, obtaining a range of 618-1430 cells per patient, of which 190-968 were CD34+ (a stem cell specific marker present in AML circulating cells 5). Clustering cells into clonal hierarchies (that means, to track the relations of descendance between the different clones) reveal the order in which mutations (either nuclear genomic mutations and/or mitochondrial mutations) have been acquired.
However, the difficulty arises in the pre-LSC condition. Although there are known the prevalence of some pre-leukemia genetic mutations in the serine and arginine rich splicing factor (2SRSF2) or the present in the DNA methyltransferase 3A (DNMT3A) genes, there are other mutations with lower expression that could be missed. For these ones, the team seek the trace of mitochondrial mutations (as mitochondria divides from “mother” cell to “daughter” cell). This strategy was revealed very useful to track cellular differentiation states and gene expression patterns associated with pre-leukemic and leukemic mutations. Therefore, refining the clonal hierarchies together with nuclear mutations the researchers found that pre-leukemic mutations were significantly associated with distinct sets of well-covered mitochondrial variants. They found a highly significant association of the SRSF2 P95H mutation with the leukemic and pre-leukemic subclones and DNMT3A mutation associated with an unrelated mutation in the RPL3 gene. MutaSeq data allowed the detection and comparison of gene expression between clones differing only in a single mutation. They found that DNMT3A mutations were the most common cause of benign clonal expansions of healthy stem cells in individuals of advanced age. Also, mutations in the multifunctional ribonucleoprotein 1 (NPM1) that frequently occur concomitantly with pre-leukemic DNMT3A mutations could be responsible of up to 30% of AML.
Finally, Velten and collaborators compared gene expression between pre-LSCs and HSCs in order to identify potential markers or drug targets that could be present in all pre-LSC, but not in HSCs to obtain preclinical targets to attack to the development of AML in its roots. This strategy leads to identify drug targets that might not have been previously tested in the right context. The results of this work could be envisaged to identify in a future cancer stem cell specific personalized drug targets, improving thus the efficiency of and reduce the side effects of current treatments.
References
- Yosep Chong, Nishant Thakur, Ji Young Lee, Gyoyeon Hwang, Myungjin Choi, Yejin Kim, Hwanjo Yu, Mee Yon Cho. Diagnosis prediction of tumours of unknown origin using ImmunoGenius, a machine learning-based expert system for immunohistochemistry profile interpretation. Diagn Pathol. 2021 Mar 11;16(1):19. doi: 10.1186/s13000-021-01081-8. PMID: 33706755 ↩
- Shelby M Knoche, Alaina C Larson, Bailee H Sliker, Brittany J Poelaert, Joyce C Solheim. The role of tumor heterogeneity in immune-tumor interactions. Cancer Metastasis Rev. 2021 Mar 8. doi: 10.1007/s10555-021-09957-3. PMID: 33682030 ↩
- Lars Velten, Benjamin A. Story, Pablo Hernández-Malmierca, Simon Raffel, Daniel R. Leonce, Jennifer Milbank, Malte Paulsen, Aykut Demir, Chelsea Szu-Tu, Robert Frömel, Christoph Lutz, Daniel Nowak, Johann-Christoph Jann, Caroline Pabst, Tobias Boch, Wolf-Karsten Hofmann, Carsten Müller-Tidow, Andreas Trumpp, Simon Haas & Lars M. Steinmetz. Identification of leukemic and pre-leukemic stem cells by clonal tracking from single-cell transcriptomics. Nature Communications 2021 Mar 1;12(1):1366. doi: 10.1038/s41467-021-21650-1. PMID: 33649320 ↩
- M Ryan Corces-Zimmerman, Wan-Jen Hong, Irving L Weissman, Bruno C Medeiros, Ravindra Majeti. Preleukemic mutations in human acute myeloid leukemia affect epigenetic regulators and persist in remission. Proc Natl Acad Sci U S A. 2014 Feb 18;111(7):2548-53. doi: 10.1073/pnas.1324297111. PMID: 24550281 ↩
- D Marisavljevic, N Kraguljac-Kurtovic. Biological implications of circulating CD34(+) cells in myelodysplastic syndromes. J BUON. Oct-Dec 2010;15(4):753-7. PMID: 21229641 ↩
1 comment
[…] da? Ezinbestekoa da erantzuna topatzea eta, horretarako, oinarrizko zientzia behar-beharrezkoa. Dr. Jekyll and Mr. Hyde: what changes in a stem cell to become malignant? Por José R. […]