Machine learning to understand water’s liquid phases
Water has puzzled scientists for decades. For the last 30 years or so, they have theorized that when cooled down to a very low temperature like -100 ºC, water might be able to separate into two liquid phases of different densities. Like oil and water, these phases don’t mix and may help explain some of water’s other strange behaviour, like how it becomes less dense as it cools.
It’s almost impossible to study this phenomenon in a lab, though, because water crystallizes into ice so quickly at such low temperatures. Now, new research 1 uses machine learning models to better understand water’s phase changes, opening more avenues for a better theoretical understanding of various substances. With this technique, the researchers found strong computational evidence in support of water’s liquid-liquid transition that can be applied to real-world systems that use water to operate.
Simulating how water intereacts
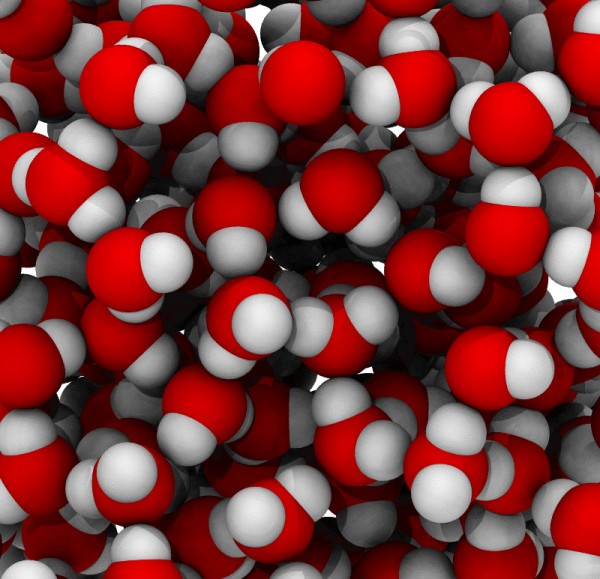
To better understand how water interacts, the researchers used supercomputers to ran molecular simulations based on very detailed quantum chemistry calculations that are trying to be as close as possible to the real physics and physical chemistry of water. These simulations work as a virtual microscope, you could zoom in all the way down to the level of the individual molecules and watch them move and interact in real time.
The researchers analysed how the molecules move and characterized the liquid structure at different water temperatures and pressures, mimicking the phase separation between the high and low-density liquids. They collected extensive data — running some simulations for up to a year — and continued to fine-tune their algorithms for more accurate results.
Even a decade ago, running such long and detailed simulations wouldn’t have been possible, but machine learning today offered a shortcut. The researchers used a machine learning algorithm that calculated the energy of how water molecules interact with each other. This model performed the calculation significantly faster than traditional techniques, allowing the simulations to progress much more efficiently.
Machine learning isn’t perfect, so these long simulations also improved the accuracy of the predictions. The researchers were careful to test their predictions with different types of simulation algorithms. If multiple simulations gave similar results, then it validated their accuracy.
Water on Earth and beyond
Some of the conditions the researchers tested were extremes that probably don’t exist on Earth directly, but potentially could be present in various water environments of the solar system, from the oceans of Europa to water in the centre of comets. Yet these findings could also help researchers better explain and predict water’s strange and complex physical chemistry, informing water’s use in industrial processes, developing better climate models, and more.
The work is even more generalizable. Water is a well-studied research area, but this methodology could be expanded to other difficult-to-simulate materials like polymers, or complex phenomena like chemical reactions.
More on the subject:
Using machine learning to discover new metallic glasses
Neutrons reveal how water dipoles relax
References
- T.E. Gartner, III, P.M. Piaggi, R. Car, A.Z. Panagiotopoulos, P.G. Debenedetti (2022) Liquid-liquid transition in water from first principles Phys. Rev. Lett. doi: 10.1103/PhysRevLett.129.255702 ↩