Using machine learning to discover new metallic glasses
Metallic glasses are a unique class of materials whose properties combine some of the irreconcilable properties of both metals and polymers. They consist of a combination of metals which, in the solid state, present an amorphous atomic structure, in contrast to common metal alloys, which present a highly ordered microscopic pattern. This lack of crystalline order in metallic glasses leads to new exciting functionalities. For instance, the absence of grain boundaries (the interface between crystals) avoids crack and corrosion propagation, making them stronger and less brittle than many other metals and alloys, perfect for structural applications. Moreover, like every other glass, they soften and flow when heated, allowing the use of inexpensive processing techniques usually applied to plastics, such as injection molding. Unlike plastics, however, they are good thermal and electrical conductors.
But, how can an amorphous structure be achieved in metal alloys? We know that all metals crystallize when solidifying from the molten state. In fact, that is their most remarkable characteristic. However, under certain circumstances, we can make crystallization quite difficult for them. Imagine that once in the molten state, a mix of different metal atoms is cooled so quickly that the amorphous, unordered microstructure characteristic of liquids remains “frozen” in the solid state. Or that this mix of metals combines so many different atomic sizes that it makes it really hard for them to accommodate in an ordered, repeatable pattern. That’s how metallic glasses are obtained, and that’s why they are categorized as kinetically stabilized materials.
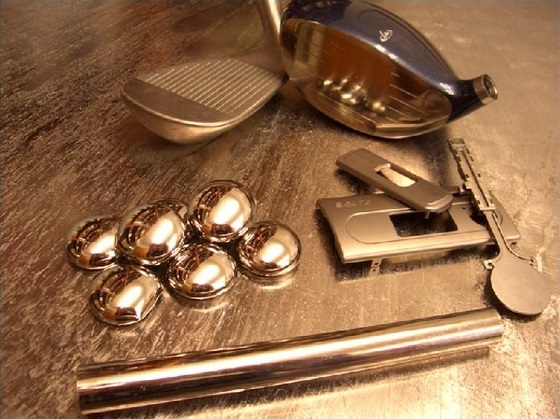
In the last few decades, a good number of metallic glasses have been produced. The first one was created at Caltech back in the 60’s, and it was a gold-silicon alloy (Au75Si25). Since then, several multicomponent alloys have proved suitable to become metallic glasses, and metals such as palladium, nickel, lanthanum, copper or zirconium are common ingredients of these formulations. However, a simple glance at the periodic table makes us aware of the huge number of potential materials resulting from combinations of two, three or more metals. In addition, the ability of these combinations to form metallic glasses depends on complex factors, including thermodynamic parameters, geometric factors and atomic number fractions, which will be different for each combination and synthesis path chosen. As of yet, there is not a universal predictor of glass-forming metallic combinations.
Conventional materials discovery based on trial and error experimentation takes very long time periods, even using automation and robotics in the lab (the so-called high-throughput screening method). As an example, searching the 3-component combinatorial space for 30 of the most common elements in metallic glasses would take decades of highly intense synthesis and characterization laboratory work. Recently, a multidisciplinary team from the SLAC National Accelerator Laboratory, NIST and Northwestern University in the US has come up with a good example of the new paradigm in materials discovery1. They have applied a machine learning model to autonomously guide high-throughput experiments in the search for new metallic glasses in the cobalt-vanadium-zirconium (Co-V-Zr) ternary system.
The machine learning approach fits perfectly in this kind of problem. Machine learning algorithms can start running with any training dataset, operate with limited knowledge of the underlying physics and finally they can autonomously improve themselves through subsequent iterations, finding hidden relationships not explained by physicochemical theories. In this study, the machine learning model has been first trained with observational datasets from well-known glass-forming alloys including 51 different elements from the periodic table. This data contains biased information (as it considers a very small fraction of the whole glass-forming space), but still is a great guide to start with. Once the training set has been selected, some cross-validation tests are carried out, in order to prove the classification performance of the algorithm. Then, a first-generation of predictions are obtained, showing the likelihood of any Co-V-Zr combination becoming an amorphous material. The picture on the left in the figure below shows this likelihood in the Co-V-Zr space, where yellow corresponds to low glass-forming chance and dark blue indicates high glass-forming chance.
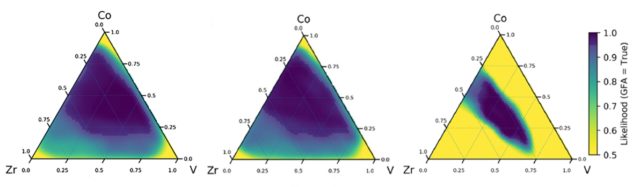
The next step towards an accurate machine learning model will be to incorporate the knowledge provided by physicochemical theories on metallic glasses to these first machine learning predictions, which were based only on the initial training dataset. The result can be observed in the middle picture above. As we can appreciate, the results are not so different from those in the left picture. Are physicochemical theories no longer relevant in the world of Artificial Intelligence? Of course they are relevant. Little could we grasp of the physical world without a deep understanding of its underlying physics. What this result emphasizes is how a powerful tool machine learning is when looking for patterns in high-dimensional data, even with scarce knowledge of theoretical relationships. Besides, the more robust the physicochemical theories are on a particular phenomenon, the bigger will be their impact on machine learning models.
Once we have this machine learning algorithm, which incorporates both experimental and theoretical knowledge, the next step is taken in the lab, through the high-throughput synthesis and characterization of ideally several hundreds of the most likely glass-forming alloys. The resulting experimental data will be used to feed again the algorithm, acting as a second training dataset in an iterative way, as depicted in figure 3. The exceptional value of this new data is that it doesn’t neglect negative results. Our first experimental dataset was obtained from 50 years of studies on metallic alloys which indeed could be synthesized as metallic glasses, excluding the invaluable knowledge provided by those compositions which actually didn’t deliver a glass-forming material. However, by incorporating machine learning to the discovery process, the algorithm can learn from negative results as well as from positive results, considerably accelerating and improving the pace of discovery.
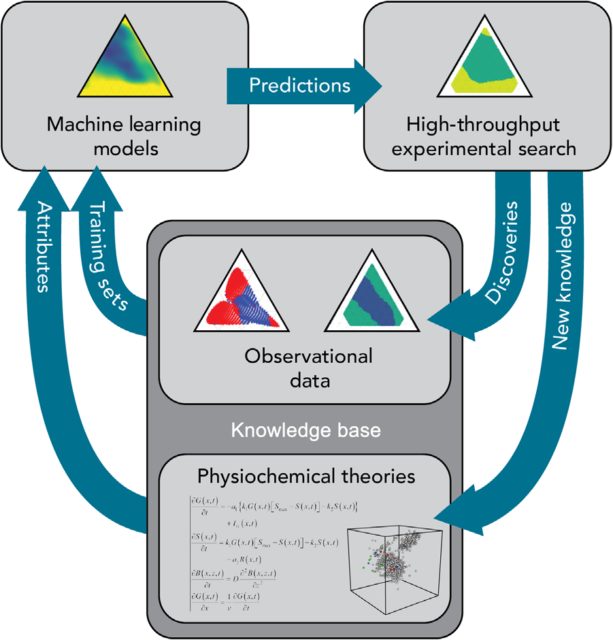
The predictions provided by the second iteration of the machine learning algorithm, which incorporates results from high-throughput experimentation, are depicted in the right image in figure 2. We can observed how the glass-forming region is now more accurately defined, considerably reducing the number of likely glass-forming combinations. The great advantage of the machine learning method is that we can iterate this cycle as many times as we like by simply feeding the algorithm with new data.
Without any doubt, the use of machine learning in materials discovery will surely increase in the near future. The demanding time periods, resources and cost derived from conventional experimentation will become obsolete as soon as recent innovations in artificial intelligence, computation and digitization are fully incorporated into Materials Science routines. The limitation will no longer be the lack of time or resources, but our ability to process and interpret large amounts of data. We are just looking at the beginning of it, and it will be exciting.
Reference:
1 Ren F. et al, Accelerated discovery of metallic glasses through iteration of machine learning and high-throughput experiments, Science Advances, 2018, Vol 4, nº 4, eaaq1566. DOI: 10.1126/sciadv.aaq1566