Status of mooring systems for floating turbines using autoencoders
Floating offshore wind (FOW) is one of the most rapidly growing forms of green power. As of 2021 though, there were only 113 MW in operation in Europe, although this figure is predicted to triple by the end of 2024, and escalate up to 10 GW by 2030 and 150 GW by 2050. The current share of floating offshore wind, however, is residual if compared to bottom-fixed offshore and onshore wind, whose global operating capacities stand at over 35 and 700 GW, respectively.
Controversy around onshore wind turbines’ noise and visual pollution, as well as potential for higher energy production, has encouraged governments and institutions to issue challenging plans for further development in the offshore sector. Spain, Norway, South Korea, Ireland and the UK are only a few examples of countries already investing heavily in floating wind projects, some of which are estimated to provide a total capacity over 2 GW once completed. Major oil and gas companies are also applying their knowledge of floating offshore technologies to wind power, partially aiming at shifting their public perception heading towards a more sustainable world.
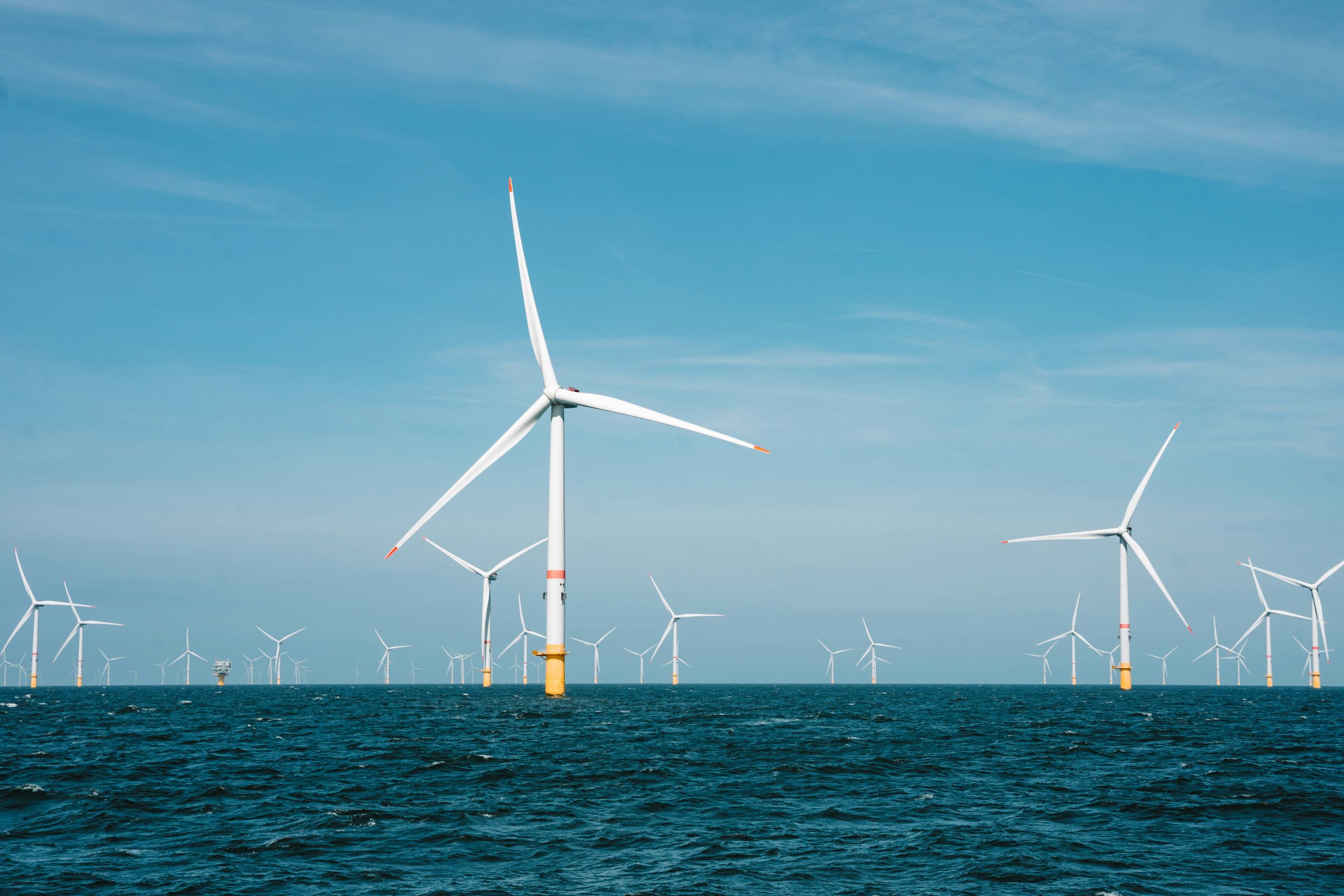
Because of their reduced noise and visual impact, floating offshore wind turbines (FOWT) are designed to be larger than their onshore counterparts. This means that their swept area is also larger, in locations where wind is already faster and smoother. All of these factors contribute to higher power output. However, excessive costs still keep floating wind farms unprofitable. Although rapidly decreasing, the levelled cost of energy of floating offshore wind varies between €90/MWh and €120/MWh, that is, more than double the cost of fixed offshore wind power. Approximately one third of these costs are due to operation and maintenance and other associated activities. New technologies, such as remotely operated vehicles, unmanned aerial vehicles, and machine learning algorithms, become essential to automate inspection and maintenance tasks, thus reducing operating costs and ultimately making FOW a commercially viable business.
In this vein, a team of researchers now proposes 1 a method for the structural health monitoring (SHM) of floating wind turbine mooring lines. These systems are one of the most expensive components of FOWTs, and are much less studied than other subsystems, such as their towers or blades.
The researchers formulate an inverse problem, in which samples of measurements from the dynamics of the floating platform are used to train a model, which can then make predictions of the structural health status of mooring lines. Solving inverse problems allows for the early identification of damage. This is critical for SHM purposes, as late notification and maintenance lead to performance losses and increased downtime. These factors crucially affect the overall budget of a FOW project, and thus need to be optimized.
Deep learning (DL) algorithms are among the most popular methods for solving forecasting and monitoring problems. These models are usually represented by deep neural networks, and have been widely used for mooring system design and SHM because of their potential to approximate complex, highly non-linear functions that go beyond the capabilities of simpler, more traditional approaches. After training, DL algorithms can make instant predictions of the health status of a system, which is crucial in the context of damage detection.
The authors detect mooring failure by implementing an autoencoder whose inputs are time and frequency-domain statistics extracted from the floater’s response in six degrees of freedom. This approach, commonly referred to as Frequency Domain Decomposition, allows for a computationally efficient analysis, as the scientists describe the structural integrity of the mooring system using a reduced set of easily measurable variables with known physical meaning.
The main novelty of this study is the deep autoencoder, designed to be fed by a reduced set of physically meaningful modal inputs describing the response of the floater. This type of network allows identifying faults under any combination of environmental conditions, and detect mooring line damage at an early stage. This is achieved by building a set of faulty mooring systems with different severities, which are defined by a set of continuous damage coefficients. These coefficients can take any value in the interval [0, 1], and are sampled for each data point using normal distributions centred around zero. This leads to a training set containing mostly undamaged and mildly degraded samples. In that way, the resulting model, whose architecture was optimized using automated machine learning techniques, is capable of detecting low-severity damages, common in early-stage operation, with a much higher rate of accuracy. The network is also capable of predicting more than one type of failure occurring simultaneously, thus increasing its potential practical applicability.
In this initial study, degradation is restricted to only two forms: biological fouling and anchor shifts. Still, the proposed algorithm is capable of detecting mild anomalies, with correlation coefficients up to 98.51% and 99.16%, respectively. These results are encouraging for the continuous health monitoring of FOWT mooring systems using easily measurable quantities to plan preventive maintenance actions adequately.
Author: César Tomé López is a science writer and the editor of Mapping Ignorance
Disclaimer: Parts of this article might have been copied verbatim or almost verbatim from the referenced paper.
References
- N. Gorostidi, D. Pardo, V. Nava (2023) Diagnosis of the health status of mooring systems for floating offshore wind turbines using autoencoders Ocean Engineering doi: 10.1016/j.oceaneng.2023.115862 ↩