2D brain mapping: gene expression and spatial location
Let’s try a little game. If I’d show you only parts of a famous building: a column, part of a wall, the ceiling…Would you be able to tell me which building it is I am telling you about? Most likely not. With these elements you could still doubt whether I am talking about an old Greek temple or the White House. To be able to tell with certainty you’d need some spatial information like where’s the building.
Now, let’s move inside your brain. In it there are many different types of neurons, and each of them is characterized by the expression of a different set of genes, even if some of those genes might be expressed by more than one type of neuron (as if two buildings in the world would have the same type of columns) and the best way to tell them apart is by having spatial information about their whereabouts in the brain.
Can we do that? It seems that now we can, at least according to a new paper by Grange et al. 1.
In recent years the advent of high throughput technologies has allowed for developments like the Allen Brain Atlas (ABA). A spatial gene expression map of the mouse brain for around 20.000 genes. One of its shortcomings is that it cannot distinguish between cell types. If two genes appeared colocalizing in the same brain area it’d be hard to tell if they’re both expressed by the same cell or there are 2 different cell types. On the other hand, another kind of approach allows to read out the full genetic profile of a certain type of brain cell, say an excitatory neuron or an astrocyte, but that’s all. What the authors of this paper did was to combine those two approaches into a model so that given a certain gene profile for a cell it would be possible to find a spatial location for it within the brain.
The first thing they did was to correlate the transcriptome of each of 64 distinct brain cell types to the gene expression data of the ABA at each voxel – a voxel being a unit in 3D space, in this case of 200 microns-. In some cases, the correlation pattern observed corresponded to that of the region from which the cells where extracted. For example, cortical pyramidal neurons were mostly correlated with the cerebral cortex. However these correlations are insufficient to tell whether a cell will be sitting at a certain brain location since they say nothing about cell type density. Therefore the need for a model based on the transcriptome data as well as the spatial density of cell types at each voxel. To do so for each cell type they ranked with higher values those brain areas in the ABA that contributed more to its correlation and density profile.
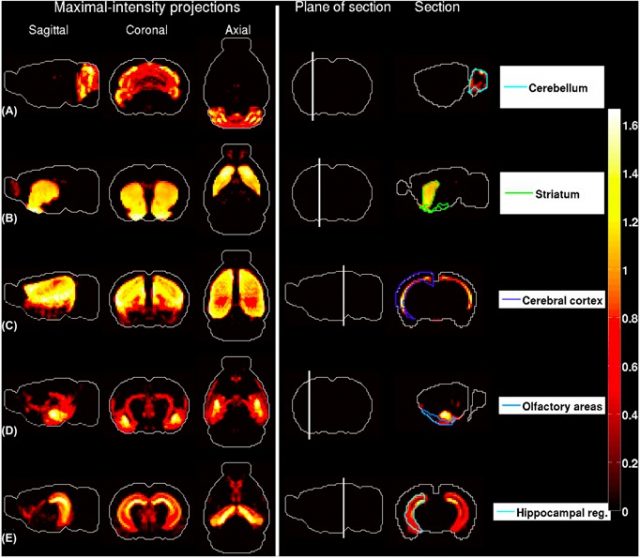
the region in the ABA with largest contribution to the density profile. The plane of section is depicted in the fourth column. (A) Granule cells extracted from the cerebellar cortex . (B) Medium spiny neurons extracted from the striatum . (C) Pyramidal neurons extracted from layers 5–6 of cingulate cortex. (D) Pyramidal neurons extracted from the amygdala. (E) Pyramidal neuronsextracted from the hippocampus. | Credit: Grange et al (2014)
In general, as a result from this model they could observe a large degree of left-right symmetry (even if the ABA dataset is not complete for all regions). They also found distinct spatially nonhomogeneus patterns for the predicted densities of 4 cerebellar cell types. Another interesting finding was that the densities of very similar cell types are negatively correlated and therefore for the model to work they should be taken together.
To assess the robustness of their model, they performed a series of tests. First they removed those genome profiling datasets suspect of contamination, and saw that except for one cell type that yielded weird results the general cell type densities remained unchanged. Then they compensated for potential cross-hybridization of microarray data and underrepresentation of certain cell types. When the problem is missing genes they tested random subsampling of 200 genes at a time to get a ranking of the cell types that were more impervious to the missing genes. So, for instance, among the top 15 cell types are pyramidal neurons.
Once proved their model is robust and a good predictor of spatial location of cell types they also characterized the probability of colocalizing genes of being part of the same biochemical path, getting a higher than chance probability. Which is not surprising, really.
In the discussion the authors are aware of the issue of the ABA being single time snapshot maps while the transcriptome data might vary since expression patterns change during development and the different states of brain activation. Which I find a mayor problem. However, they point out that the cell type densities predicted allow for the emergence of brain regions, even despite the issues with the incomplete datasets, so they are confident of the validity of their method to annotate cell transcriptomes to brain regions, at least for the mouse. For the human we’ll have to wait since the ABA map is far from complete and samples are scarce.
So, to wrap up Grange et al. have created a mathematical model based on experimental data coming from both single cell transcription studies and the gene mapping Allen Brain Atlas to be able to spatially map a cell type based on its genetic profile. Even though the method still has some issues that need improving it’ll be a very useful tool for brain scientists. Now it will be easier to link function to cell types and therefore to gene networks in health and disease since we know -more or less- from fMRI and other studies the functionality of brain areas so we will be able to add one plus one. Another step forward in this long run towards knowledge in neuroscience.
References
- Grange P., Bohland J.W., Okaty B.W., Sugino K., Bokil H., Nelson S.B., Ng L., Hawrylycz M. & Mitra P.P. (2014). Cell-type-based model explaining coexpression patterns of genes in the brain, Proceedings of the National Academy of Sciences, 111 (14) 5397-5402. DOI: 10.1073/pnas.1312098111 ↩