From gas to cash. The unexpected applications of statistical mechanics
Mechanics and thermodynamics are two of the main pillars of physics. Mechanics, the branch of physics that studies movement, is considered to be well established since the publication of Isaac Newton’s Philosophiæ Naturalis Principia Mathematica (1687). Thermodynamics, the science dealing with heat and temperature, enjoyed its golden age in the 19th century, and was the theoretical framework that allowed the Industrial Revolution. Most of its postulates are phenomenological, being the law of ideal gases the best example of that way of proceeding (Clapeyron, 1834) as it’s derived as a combination of two phenomenological laws (Boyle’s and Charle’s laws).
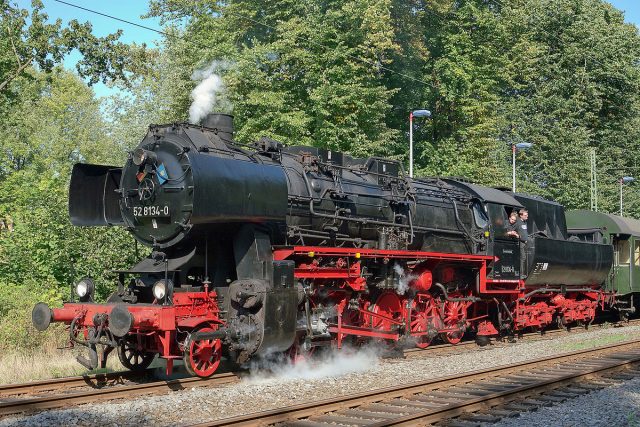
Both disciplines evolved independently, but, in the 19th century, the field of statistical mechanics emerged as a link between them. The merging path started to become clear with the development of the kinetic theory. It is unclear who the father of the idea was; some go as far as Epicurus, but a safer bet is Daniel Bernoulli, who, in his Hydrodynamica (1738) modelled a gas as a large number of solid particles in constant movement.
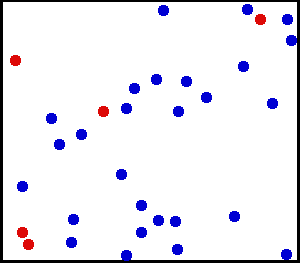
Such an apparently simple and promising way of modelling a gas encountered two main obstacles. The first one was that a gas certainly doesn’t look like a group of solid particles (in fact, the atomic nature of matter had to wait until Einstein’s work on Brownian motion (1905) was definitely settled). The second, and more important obstacle, was the difficulty of applying mechanics to such a large amount of particles. To give an estimation of that difficulty, keep in mind that a mole, which is a usual quantity in macroscopic experiments, contains more than 6 · 1023 particles. This leads to the same number of dynamical equations. Even if the equations were uncoupled, just setting the boundary conditions would be an impossible amount of work1.
The solution appeared in the form of statistics. This approach turned the problem of the huge amount of particles into an advantage. Why? Just because statistical predictions tend to gain accuracy as the samples grow. Think of a survey about voting intentions… it is straightforward that better results will be obtained if the survey is conducted on a large (and properly sampled) subset of the population. As a rule of thumb, the larger the sample, the better the predictions.
This approach required ignoring the individual mechanical properties and focusing on their averaged values. This led to surprisingly simple relationships between thermodynamic and mechanical magnitudes. For example, the temperature emerged as the average of the kinetic energy of the particles (with a proportionality constant), and the pressure as the average of the linear momentum transfer between the particles and the container walls per unit time and area.
The example of the pressure is very illustrative. Think of a crowd trying to push down a door, or a wall. If the crowd is large enough, the wall as a whole would experience an almost constant push, despite each person in the crowd is just pushing locally and for a while. Of course, if the crowd is reduced to a few people, or to a single person, the statistical approach is no longer useful. If you prefer a less violent example, think of holding an umbrella under heavy rain; if you’ve got good imagination you’ll even feel in your arm the constant extra force you need to hold the umbrella against it.
Once again, an apparently elaborated mathematical concept (as the average) turns out to have a very straightforward physical interpretation under some circumstances. This led us to a new fascinating question: What is the pressure (or the temperature) of a single particle? The answer is that, despite the fact that defining it is feasible, it has no physical meaning… or at least not the same straightforward meaning as in the example with lots of particles.
Pressure or temperature are extremely simple examples of the so-called emerging properties: properties that only make sense under a big amount of interactions, but apparently don’t exist when the interactions are studied individually. As Aristotle said: “The whole is greater than the sum of its parts”. As we will see later, emergent properties are in the core of the field of complex systems.
One of the remarkable results of the kinetic theory is that the law of ideal gases can be derived from it. That is, the laws of movement can explain a thermodynamic law.
Kinetic theory was one of the touchstones of the new field of statistical mechanics. Since its foundations were developed in the second half of the 19th century (mainly due to Clausius, Maxwell and Boltzmann), statistical mechanics harvested one success after another.
The details of the theory of statistical mechanics are far beyond the scope of this article. As a summary, I’ll just say that problems in classical statistical mechanics usually have these elements:
-
A huge amount of particles.
-
Some common well known dynamic properties (for example, all particles can be inside a container, have the same mass, or be under the influence of a gravitational field).
-
Some common partially-unknown dynamic properties, introduced via probability density functions (for example, the kinetic energy distribution given the temperature).
Or, stated in a much simpler way:
-
A description of the system in terms of its components.
-
What we know about the components.
-
What we don’t know so well.
Of course, there is a wide range of phenomena that can be naturally modelled as a system whose behavior is only partially known. And in such cases, the methods of statistical mechanics become a useful tool.
For example, think of the stock market. A very simple model of it can be fitted into our scheme as follows:
-
The buyers and sellers (the individual elements).
-
The fact that all of them want to maximize their earnings (the common “driving force”).
-
The random fluctuations and the weight of bad decisions (the unknown, the random noise).
Most of these “exotic” applications of statistical mechanics have very serious problems. Being the following the most important:
-
Usually, the deterministic part of our problem (the Hamiltonian or driving force) of our elements is not clearly defined.
-
The systems are, by far, smaller than the usual systems in statistical mechanics. So the statistical approach loses a lot of accuracy when compared with classical examples, with moles and moles of elements.
Despite these and other flaws, even such an oversimplified description is still an interesting approach to this kind of problems. In fact, statistical mechanics was the seed for the methods of the so-called complex systems science. Complex systems science is a subfield halfway between physics and applied mathematics, which evolved mostly (but not only) from statistical mechanics.
Currently, the field of complex systems covers an enormously wide range of applications in almost every field of knowledge (such as ecology 1, demography 2, economy 3 and, going back to our example of the raging crowd, even riot control 4 and law-enforcement 5).
Bibliography:
W. Greiner, L. Neise, H. Stöcker. Thermodynamics and statistical mechanics. New York. Springer – Verlag. 1997. ISBN 978-1-4612-0827-3
J. Aguilar. Curso de termodinámica. Pearson Educación. ISBN 9788420513829.
Note:
1 Just for storing this data, assuming 2 bytes per number and 6 numbers per particle (position and momentum), will be required about 1016 Gb. This is roughly equivalent to 10.000 computers per person in the world. Not to talk about the horrible task of typing it all.
References
- Garcia-Algarra, Galeano, Pastor, Iriondo, Ramasco. Rethinking the logistic approach for population dynamics of mutualistic interactions. Journal of Theoretical Biology (2013). ArXiv ID: 1305.5411 ↩
- Castellano, Castellano, Fortunato et al. Statistical physics of social dynamics. Reviews of Modern Physics (2007). ArXiv ID: 0710.3256 ↩
- Yakovenko. (2012). Applications of statistical mechanics to economics: Entropic origin of the probability distributions of money, income, and energy consumption. ArXiv ID: 1204.6483. ↩
- Helbing, Johansson, Al-Abideen. Crowd turbulence: the physics of crowd disasters. (2007) ArXiv ID: 0708.3339 ↩
- D’Orsogna, Perc. Statistical physics of crime: A review. (2000). ArXiv ID: 1411.1743 ↩