How to scale up a pilot program
Economics needs theory, lab experiments, historical data and field evidence. Pilot programs and randomized controlled trials are particularly credible from the point of view of internal validity. However, the results of these “proof-of-concept” studies do not necessarily extend beyond the context in which they where implemented or scale up if they are generalized.
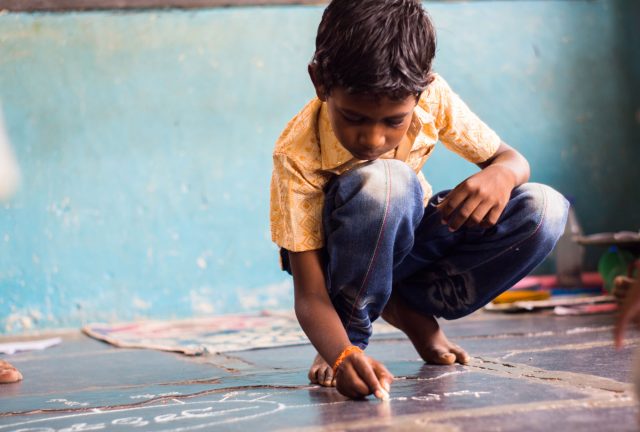
Banerjee et al. (2017) 1 study six main challenges in drawing conclusions for localized random controlled trials: market equilibrium effects, spillovers, political reactions, context dependence, randomization or site-selection bias, and piloting bias. Then, they document the overcoming of some of these challenges using an example of a successful intervention that started by a nongovernment organization in a few slums, and was developed to a policy implemented at scale by state governments in India. Here we present a summary of their work using selected paragraphs from the article.
The challenges
1. Market Equilibrium Effects
The first challenge should already be familiar to economists. A small-scale implementation may have only partial equilibrium consequences, whereas a large-scale one may affect the general equilibrium. As a result, the outcomes of a small-scale program may under or over estimate the outcomes of generalizing it. For example, granting a scholarship to a small group of students may have a big effect on the group, but a generalization of the scholarships will increase the educational attainment across the entire population, thus decreasing the overall return to education (Heckman et al., 1998 2).
As another illustration, consider the low impact of microcredits on beneficiaries according to randomized control trials (Barnejee et al., 2105 3 review this literature). However, the sudden collapse of microcredit in Andhra Pradesh, India, due to political and not economic reasons, caused a large negative effect on the communities where it was used. Although the mechanism is not fully understood, it seems that the microcredit has a multiplier effect where it is implemented, something that would not have been captured in the micro data.
2. Spillover Effects
Many treatments have spillovers on neighboring units, which implies that those units are not ideal control groups. Some spillovers are related to the technology. For example, intestinal worms are contagious, so if a child is dewormed, this will affect her neighbor. Other channels of spillover are informational: when a new technology is introduced (like a long-lasting insecticide-treated bed-net), the first people who are exposed to it may not take it up or use it properly.
3. Political Reactions
Political reactions, including either resistance to or support for a program, may vary as programs scale up. Corrupt officials may be more likely to become interested in stealing from programs once they reach a certain size (Deaton 2010 4).
4. Context Dependence
Evaluations are typically conducted in a few (carefully chosen) locations, with specific organizations. Would results extend in a different setting (even within the same country)? Would the results depend on some observed or unobserved characteristics of the location where the intervention was carried out?
5. Randomization or Site-Selection Bias
Organizations or individuals who agree to participate in an early experiment may be different from the rest of the population. This may be because the willing partners are particularly competent and motivated, because those who are more likely to benefit are also more likely to be treated, or because the organization, knowing it will be evaluated, chooses a location or a subgroup where effects are particularly large.
6. Piloting Bias/Implementation Challenges
A large-scale program will inevitably be run by a large-scale bureaucracy. The intense monitoring that is possible in a pilot may no longer be feasible when that happens, or may require a special effort.
A Successful Scale-up: Teaching at the Right Level
Pratham, an Indian nongovernmental organization, designed a deceptively simple approach, which has come to be called “teaching at the right level”. The basic idea is to group children, for some period of the day or part of the school year, not according to their age, but according to what they know.
From Bombay Slums to 33 Million Children
The partnership between researchers and Pratham started with a “proof of concept” randomized controlled trial of Pratham’s Balsakhi Program in the cities of Vadodara and Mumbai, conducted in 2001–2004 (Banerjee et al. 2007 5). Their learning levels increased by 0.28 standard deviations.
Pratham next took this approach from the relatively prosperous urban centers in West India into rural areas, where they were forced to rely largely on volunteers rather than paid teachers. To facilitate this change, the pedagogy became more structured and more formal, with an emphasis on frequent testing. A new randomized evaluation was therefore launched to test the volunteer-based model in the much more challenging context of rural North India.
The results were very positive, bur also revealed new challenges: Volunteers’ enthusiasm decreased, classes ended prematurely, and only 12% of eligible students were treated, leaving behind students from the bottom end disproportionately.
A First Attempt to Scale-Up with Government
Starting in 2008, Pratham and the Abdul Latif Jameel Poverty Action Lab embarked on a series of new evaluations to test Pratham’s approach when integrated with the government school system.
Different implementations were conducted to determine the causes of success or failure. In the interventions that provided the program with material or material plus training they found no effect. When volunteers were added to the program, they caused a positive impact in Bihar, but not in Uttarakhand, the two states where the study was conducted. At first glance, it seemed that the failure of schools to utilize the volunteers as intended (they were made part of the school team) might be the reason why the Uttarakhand intervention did not work. However, a careful analysis showed that the reason was not the distinction between volunteers and government schoolteachers, but between personnel that incorporated the targeted teaching aspect and those who did not. In other interventions, where the program was implemented by schoolteachers during summer camps, it provided positive results. Why don’t they do so during regular school day?
Getting Teachers to Take the Intervention Seriously
First, all efforts were made to emphasize that the program was fully supported and implemented by the government, rather than an external entity. Second, the program was implemented during a dedicated hour during the school day. This change sent a signal that the intervention was government-mandated, broke the status quo inertia of routinely following the curriculum, and made it easier to observe compliance. Third, during the extra hour children were reassigned and physically moved to classrooms based on levels. This removed teacher discretion on whether to group children by achievement.
This new version of the program was evaluated in the school year 2012–2013 in 400 schools, out of which 200 received the program. This time the results were positive.
Still, in areas where this was difficult to implement because the teaching culture is very weak, Pratham, with the permission of the district administration, developed the in-school “Learning Camps” model using volunteers.
It took five randomized control trials and several years to traverse the distance from a concept to a policy that actually could be successful on a large scale, benefiting millions of children.
References
- Banerjee, A.; Banerji, R.; Berry, J.; Duflo, E.; Kannan, H.; Mukerji, S.; Shotland, M., and Walton, M. 2017. From Proof of Concept to Scalable Policies: Challenges and Solutions, with an Application. Journal of Economic Perspectives 31(4), 73–102. ↩
- Heckman, J. J.; Lochner, L., and Taber, C. 1998. Explaining Rising Wage Inequality: Explorations with a Dynamic General Equilibrium Model of Labor Earnings with Heterogeneous Agents. Review of Economic Dynamics 1, 1–58. ↩
- Banerjee, A.; Karlan, D.; and Zinman, J. 2015. Six Randomized Evaluations of Microcredit: Introduction and Further Steps. American Economic Journal: Applied Economics 7(1), 1–21. ↩
- Deaton, A. 2010. Instruments, Randomization, and Learning about Development. Journal of Economic Literature 48(2), 424–55. ↩
- Banerjee, A.; Cole, S.; Duflo, E., and Linden, L. 2007. Remedying Education: Evidence from Two Randomized Experiments in India. Quarterly Journal of Economics 122(3), 1235–64. ↩
1 comment
Dear Dr,
Warm greetings from Addis Ababa Ethiopia!
I read your article on “How to scale-up a pilot program” to benefit others in the near future. Your view in the context of economic equilibrium is very sensitive and of course needs further studies. I believed that there is no full utilization of scare productive resources ( Full Employment). For me, both political and corruption challenges to scale up a given pilot project/program is top one. I also very interested in to work applied research in this topic.
In general, it is very interesting and useful article for me.
Thank you for your contribution!