Artificial intelligence reveals the rules of fighting
Author: Marta Iglesias-Julios is currently a PhD candidate (Champalimaud Neuroscience Programme) at the Campalimaud Center for the Unknown in Lisbon (Portugal)
Science often advances through improvements in our ability to measure and observe. Just take the example of migrating storks. In the beginning, the Egyptians had a mystery: storks appeared in Africa during winter and disappeared in the summer. As explorers travelled the world, they saw the storks pop up in middle Europe during the summer months and so the idea of a migration was born. Then scientists began marking individual storks, and they discovered multiple migration routes. With modern GPS trackers, scientists can even study how many storks coordinate their joint flight and how the storks take advantage of thermal updrafts to save energy. With each advance in methods of observation came along new discoveries.
Inspired by this history, our laboratory has been interested in developing tools for improved measurement of behavior. A good understanding of animal behavior is a key component on which other fields like neuroscience, molecular biology or evolutionary biology can build on. Only when we understand the behavior can we truly study how that behavior is produced in the brain or what the survival benefits of a particular behavior are.
Behavior is a difficult thing to study because it is variable and we need to gather very large data sets before we get an accurate picture of what is going on. In the past, such data sets were often gathered by painstaking human labor: someone had to go through many hours of video data and annotate what was going on in the video at every moment. In our case, we were studying fighting in zebrafish, where a typical fight can take up to a week for a human to annotate. We wanted to automate this process with the help of AI.
Using recent advances in a field of artificial intelligence known as deep learning, we trained 1neural networks to imitate the annotations of a trained human. Once we had a trained network, a new video of a zebrafish fight could now be annotated in a matter of a few minutes rather than in a week as was previously required.
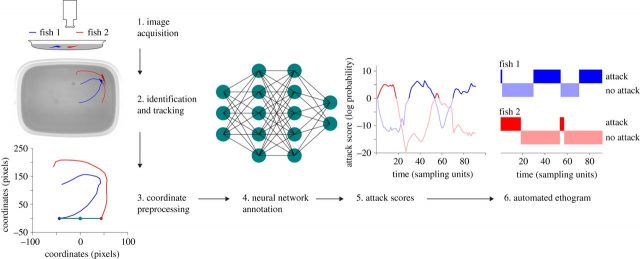
After we had completed our new high throughput measurement system, we set about using it to learn more about the rules of fighting. In particular, we wished to know more about the energy costs involved when fish are performing the various manoeuvres that make up a fight. Just like a sports coach cannot optimize an athlete’s strategy without knowing how much energy various athletic maneuvers take, it is similarly difficult to understand the logic of a fish fight if you do not know the energy costs involved in the process.
Our measurement system allowed us to keep track of the speed and acceleration patterns of fighting fish. We found that zebrafish fight at a runner’s pace. A fighting zebrafish swam almost two and a half times faster than an exploring or calmly strolling zebrafish. The zebrafish also liked to punctuate their sprints with violent flips. During one of these flips, a zebrafish can completely reverse its movement direction faster than the blink of an eye. A fighting zebrafish can complete a full body jump (a full body U-turn in space) in just 50 milliseconds- that is two times faster than one tenth of a second. A human could never pull off a maneuver this fast.
While the above findings told us that fighting was obviously a strenuous and dangerous activity, we also learned that zebrafish liked to keep things civil if they can. By analyzing how fish responded to mutual strikes and bites, we found that most of the time, the fish kept up a gentlemanly manner. Attackers often swam within striking distance of their opponent, but they rarely ever carried out the strikes. The defenders also often deliberately revealed their vulnerable sides in a provocative manner. There appeared to be a great deal of mutual posturing going on. Attackers were demonstrating their potential to strike and defenders were showing off their ability to handle a strike or a bite while the actual number of bites was kept at a minimum.
Based on these results, we can think of zebrafish fighting as an exchange of credible threats. They need to perform the energetically costly flips and rapid runs to credibly show off their skills, but they also like to keep things from escalating too far into the domain of potentially deadly bites and wrestles. While this description of a zebrafish fighting is still rather informal, we hope in the future to use our extensive quantitative data sets to carry out a full evolutionary game theory analysis of this hypothesis in order to establish to what extent this picture is accurate or not.
Our findings would not have been possible without the use of advanced measurement which enabled us to study a much greater volume of data than has previously been possible. In future studies, we hope to bring these techniques to bare on a number of different phenomena like comparing fights between different species of fish and studying how fish develop their fighting skills over time.
References
- ↩ (2018) Zebrafish aggression on the sub-second time scale: evidence for mutual motor coordination and multi-functional attack manoeuvres Royal Society Open Science doi:
1 comment
[…] Seguro que en los próximos años se publicarán muchos otros artículos con el uso del aprendizaje profundo en etología. El nuevo artículo es Andres Laan, Marta Iglesias-Julios, Gonzalo G. de Polavieja, “Zebrafish aggression on the sub-second time scale: evidence […]