Mining Wikipedia to unveil emergent interdisciplinary knowledge
Specialisation has necessarily led to the fragmentation of knowledge, creating loosely connected disciplines in which discoveries in one area are hardly known in others. This implies that the flow of knowledge is severely restricted among disciplines or even among different areas within the same discipline.
In recent decades, different approaches have been proposed to overcome this gap by means of, for example, co-occurrence, semantic models or bibliometric-based systems that use citation information to find related items. Still, interdisciplinary research lacks efficient tools for establishing quantitative connections among different disciplines (such as science, art and literature). This problem becomes even more important if we consider the amount of available knowledge, which is so large as to make it impossible for a human being to read or even access it in its entirety.
Wikipedia is one of the most impressive collective creations: millions of anonymous editors work, in a mostly non-coordinated way, to build the greatest source of knowledge that humanity has ever seen. Mining such a public knowledge database can reveal surprising relationships among elements belonging to apparently distant disciplines.
Interestingly, in addition to the explicit knowledge contained in Wikipedia articles, there is a vast amount of implicit learning that emerges from the underlying dense network of internal links that represent connections among people, ideas and works and constitutes a large conceptual network. Internal links refer to those links present in the main text of an article that connect relevant elements with other articles within Wikipedia. This giant network (~163M connections in the English version) can be converted into a directed graph and has actually been used in many studies ranging from computing semantic relatedness to natural language processing.
Now, inspired by these successful approaches and to overcome the lack of quantitative methods in interdisciplinary research, Gustavo A. Schwartz proposes 1 a non-supervised method to reveal emergent knowledge in Wikipedia using network science.
Schwartz starts from the publicly available WikiLinksGraphs datasets that contain the network of internal links (only those intentionally added by editors in the main text of the articles) for different dumps of Wikipedia. The idea is to unveil how two or more elements (concepts, people, works) are related and connected among them. Therefore, starting from some selected elements (entries of the Wikipedia he calls seeds), a subgraph (universe) is defined by taking the nearest neighbours to each seed(s).
As a proof of concept, the relationship between the works of Albert Einstein (science) and Pablo Picasso (art) at the beginning of the twentieth century is investigated. Was it a coincidence that Picasso developed Cubism at approximately the same time that Einstein published his theory of relativity? Were they answering the same questions? Were they influenced by the same people/works?
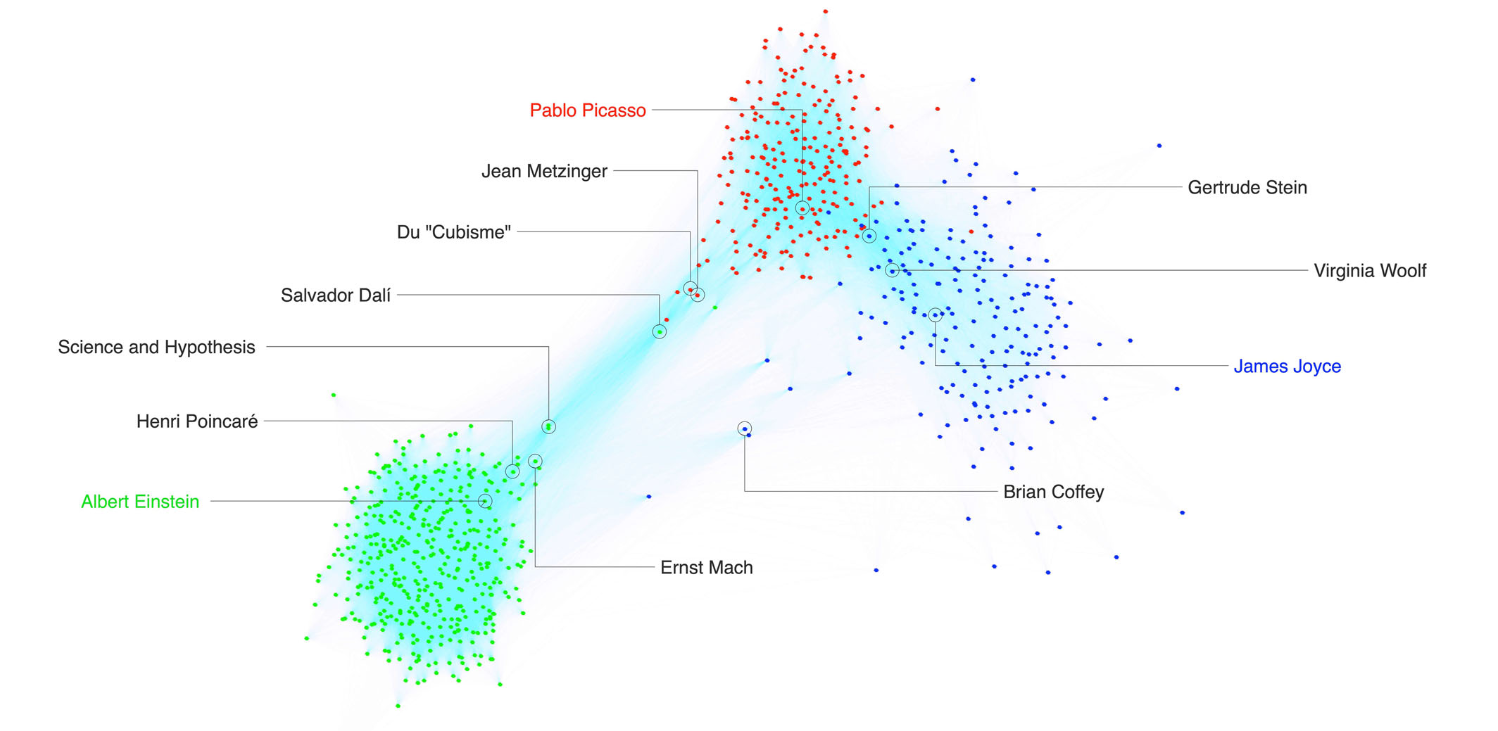
Therefore, the seeds are ‘Pablo Picasso’, ‘Albert Einstein’and ‘James Joyce’. Although the focus is on the Einstein-Picasso relationship, including Joyce allows to compare the relationships among art, science and literature, and to perform a deeper comparative analysis. Thus, based on these seeds, a universe was obtained containing 78,444 nodes and 3,159,866 edges. Then relatedness is defined and measured.
Figure 1 shows a visual representation of the universe, which constitutes a knowledge map for the relationships among Picasso-, Einstein- and Joyce-related elements. We can clearly observe three well-defined clusters corresponding to the elements most related to each seed. These clusters also account for the three domains to which each of the seeds belongs: art, science and literature. Artistic and literary domains are close and very well-connected; much more than any of them with the cluster related to Einstein. On the other hand, science-related nodes show a stronger connection with those related to art than with those in the literary domain. Schwartz quantifies these structural characteristics.
The complex networks approach proposed here shows the need to consider interdisciplinary knowledge as a whole instead of focusing on local and specific information. Moreover, it highlights
the emergence of collective knowledge that can arise from individual uncoordinated actions.
Author: César Tomé López is a science writer and the editor of Mapping Ignorance
Disclaimer: Parts of this article may be copied verbatim or almost verbatim from the referenced research paper.
References
- Schwartz, G.A. (2021) Complex networks reveal emergent interdisciplinary knowledge in Wikipedia . Humanit Soc Sci Commun doi: 10.1057/s41599-021-00801-1 ↩