AI can predict Alzheimer’s risk from brain scans
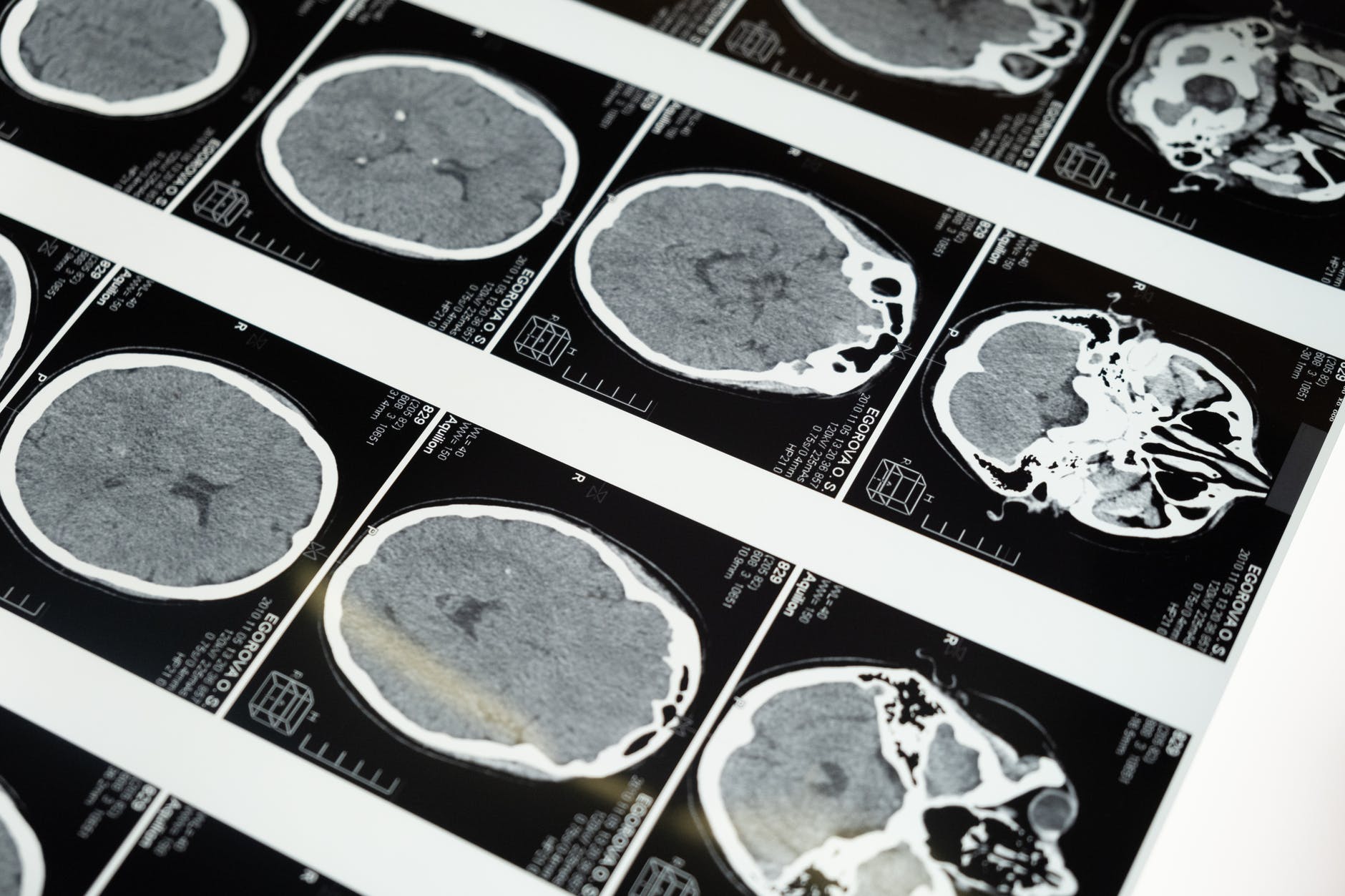
Since humans are not very good at predicting the future, even if the help of a magic ball, scientists have developed a new system based on artificial intelligence to help us predict the future risk of suffering from Alzheimer’s disease by analysing certain parameters in brain scans.
The research, recently published in the journal Diagnostics 1, provides insights into the development of such an AI system, able to recognise early signs of cognitive impairment (a previous stage to full-on Alzheimer’s) on brains scans acquired with functional magnetic resonance imaging (fMRI).
To develop their AI system, first the original brain network ResNet18 was trained on 51,443 brain scans from 138 people. The images corresponded to six distinct groups, from healthy brains to various degrees of mild cognitive impairment (MCI) and full-blown AD. Then, other 27,310 images were used to validate the algorithm. The final algorithm could identify early MCI with 99.99 percent accuracy and late MCI with 99.95 percent accuracy.
The results of this study show that this newly developed system has better accuracy, sensitivity, and specificity than older diagnostic algorithms, and remark its potential as an assisting tool for earlier detection of cognitive decline.
Another important point to make is that while MCI often precedes Alzheimer’s disease, not all people showing signs of MCI will develop the disease. The power of this tool is to allow early screening and closer follow-up for early intervention.
However, as good and helpful as machine learning algorithms are, two pairs of eyes are better than one, and the combined effort of this method plus human expertise are the ideal combination for accurate diagnosis.
References
- Odusami, Modupe, Rytis Maskeliūnas, Robertas Damaševičius, and Tomas Krilavičius (2021) Analysis of Features of Alzheimer’s Disease: Detection of Early Stage from Functional Brain Changes in Magnetic Resonance Images Using a Finetuned ResNet18 Network Diagnostics 11, no. 6: 1071. doi: 10.3390/diagnostics11061071 ↩