Can older adults anticipate words better than younger adults?
anticipate
Authors: Victoria Cano Sánchez & Patricia Fuente García, PhD candidates at the University of the Basque Country, Dept. of Linguistics and Basque Studies (UPV/EHU) and members of The Bilingual Mind Research Group (Gogo Elebiduna)
Have you ever wondered why are we able to respond and comprehend others as fast as we do? The answer to this mystery lies in a primitive mechanism that we all have as humans: the ability to anticipate the future.
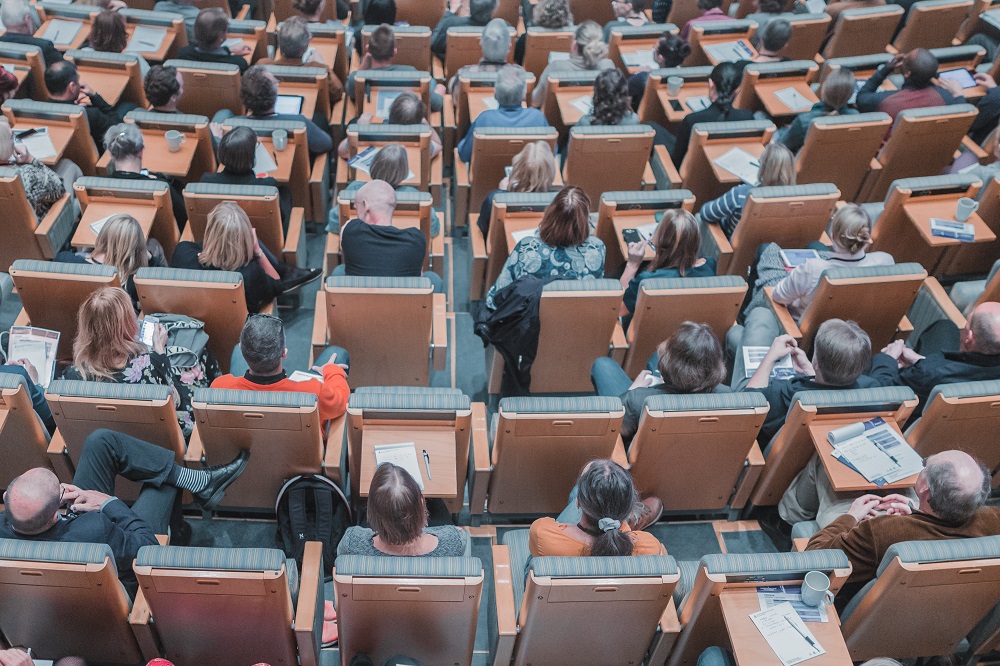
During our everyday life, it is precisely this remarkable capacity for anticipation (Bar 2007) what can make the difference between life and death, for example, in front of a predator. It allows us to rapidly anticipate a specific change in our environment, that is, a concrete danger, as it would be the presence of a predator, and prepare us to swiftly react accordingly to save our lives. Likewise, in the realm of language processing, during the communication process we are constantly exposed to a series of stimulus (e.g.: intonation, gender, volume, coarticulation, prosody, minimal pairs, etc.,) that, as speakers, we have to decode and interpret, and despite the complexity of this process, our brain adeptly accomplishes this task almost effortlessly within mere milliseconds (Federmeier, 2007; Dikker et. al., 2010).
According to some theories, that primitive predictability mechanism that humans possess would be also the secret ingredient which allows us to anticipate the forthcoming linguistic information, ultimately enabling an efficient linguistic comprehension (Kuperberg & Jaeger, 2016). That is, when we engage in a conversation, we are constantly making predictions about the upcoming words our interlocutor is about to say. Thanks to this predictive ability, we can, by means of analogies and associations, sometimes even finish their sentences. This capability relies on our expectations and our broad understanding of the world.
For example, when we come across a sentence like “I will go to the fountain to drink some …” our minds naturally and automatically predict that the next word will be “water”. Similarly, if we consider once again the previous sentence, and we say now “I will go to the fountain to…” we will also predict that the upcoming word would be “drink” and not “drinked” or “drinking”. Consequently, we have the ability to predict not only specific words, but also their grammatical forms, and this enhances, at the same time, our capacity to resolve ambiguities as we process the unfolding language. It’s quite intriguing, isn’t it? In essence, these predictions about what information we are likely to encounter in upcoming parts of the sentence or conversation (Bar, 2007; Chemairou, 2016) play a role in making our language processing smoother. They contribute significantly to our swift comprehension and the fluidity of our conversations (Pickering and Garrod, 2007), which benefits us all.
The brain, an engine of probabilistic prediction
This idea of prediction as the basic mechanism underlying language processing is not new in cognition theories. In fact, several studies (e.g. Helmholts, 1866/1962, DeLong et al., 2005; Clark, 2013) have suggested that the brain would act as “an engine of probabilistic prediction” (Lupyan & Clark, 2015, p.279), because based on previous experiences, it would be constantly generating hypotheses to contrast them against the real linguistic information we perceived.
According to this concept, it has been commonly argued in psycholinguistics that linguistic processing can be approached through two contrasting models, each differing in how they generate hypotheses based on the linguistic information they receive. On one side, we have the top-down model, which suggests that we process language starting from the broadest information, such as concepts and meaning, and gradually work towards more specific and detailed information, such as phonemes or letters. For instance, when comprehending a sentence like “The big brown dog is sleeping”, the top-down model would first grasp the overall meaning and intent of the sentence (e.g., it’s about a dog sleeping), and then delve into finer linguistic details. On the contrary, the bottom-up model contends that our processing begins with the most specific information and progressively incorporates more general elements (Lupyan & Clark, 2015; Ferreira & Chantavarin, 2018). For example, in the sentence “The big dog is sleeping”, the bottom-up model would begin with processing individual letters and sounds, building up to recognize words, and ultimately synthesizing the sentence’s overall meaning based on this detailed analysis.
However, we rather acknowledge now that it is not a matter of one model versus the other, but a dynamic and bidirectional system of information exchange. In this system, information—the predictions/hypotheses to be tested—is processed simultaneously from higher levels of representation to lower levels, and viceversa. This simultaneous processing takes into account both, background knowledge and experience-driven predictions about the intended meaning of the language we are about to hear or read, as well as the bottom-up processing where prediction errors move from lower levels to higher representational levels (Chemairou, 2016). In simpler terms, it involves moving from what we initially expect to hear or read to the actual sounds or words we encounter and perceive, all the way up to concepts and meanings, confirming or revising our initial predictions in the process.
A dynamic interplay between gains and losses
Current theories of aging argue that the accuracy of predictions is influenced by the “dynamic interplay between gains and losses” (Stine-Morrow, 2007), that is, by the “key opposing forces” of increased language experience and cognitive decline in aging (Reifegerste, 2021).
And maybe you are wondering: if the older, the wiser, does it imply that older adults can anticipate words better than their younger counterparts? Surprisingly, the answer remains unclear.
On the one hand, learning is an ongoing process that continues throughout our lives, extending beyond adulthood. Thus, with the accumulation of additional exposure and experience with language over the years (e.g. vocabulary size), our knowledge is subsequently mirrored in the formation of stronger learned associations. As a result, older adults should be capable of making more accurate, up-to-date, and refined predictions (Mani & Huettig, 2014; Harada et al., 2013). Similarly, as individuals gain more language experience, it is theorized that the activation of competing linguistic elements diminishes (Chemairou, 2016; Kukona et al., 2014). This occurs as language users become more adept foreseeing upcoming words.
However, at the same time, we know that age can be a double age-sword. As we age, we experience perceptual declines, including reductions in visual and auditory acuity (Lindenberger & Baltes, 1994). Additionally, cognitive decline, understood in terms of deterioration in the underlying neural mechanisms (Salthouse, 1996), tends to impact problem-solving abilities such as: working memory capacity, i.e the ability of holding important information for a short time on memory in order to complete complex tasks like understanding language or solving problems (Engle, 2002); processing speed, i.e. the rate at which we can execute the processing of certain operations (Salthouse, 1996); and executive control, i.e. the ability to plan, organize, initiate and monitor cognitive tasks, as well as to inhibit or shift attention as needed (Fjell et al., 2016) (Reifegerste, 2021; Fjell et al., 2016; Burke & Osborne, 2007). These age-related changes may then diminish the ability of older adults to predict upcoming words in a sentence as it unfolds over time (Huettig & Janse, 2016).
Not in vain, when discussing about cognitive decline and predictability, it’s important to understand the intricate relationship between cognitive factors and the formation of predictions, which is rooted in a three-step process.
Firstly, predictions are formed by connecting current information with past experiences, essentially comparing what we know now with what we’ve learned before and have stored in our memory (Bar, 2007). And the speed at which we do this is influenced by how quickly we can process new information and link it to our stored knowledge.
Secondly, working memory has a crucial role here, because it helps us to hold past information active in memory while taking in new information.
Lastly, executive control aids us in selecting the most relevant information from a pool of various competing options and discarding the incorrect ones.
In the end, the predictive process is extremely related to the evolution of the aforementioned cognitive factors, which we have seen are highly sensitive to the effects of aging in a way such that as we get older, they tend to decline. Consequently, this decline can potentially counterbalance the benefits of the increased language experience accumulated with age, fronting us, once again, with the two faces of the same coin: language experience vs. cognitive decline. Is there a trade-off between these two opposing forces?
In sum, in this article we have shown that prediction is a fundamental part of efficient language processing, and that age, contrary to what has been believed up to recently, is a determining factor in shaping the evolution of linguistic predictability and language processing throughout one’s life. Yet, our understanding of how these predictive mechanisms evolve with age remains limited, despite their far-reaching implications in today’s society.
In a social context where people are living longer and the populations ‘average age keeps rising, it’s essential for us to understand how our thinking abilities change as we age in order to lead healthy lives and enhance well-being throughout our lives. This knowledge not only helps us better grasp how our language processing evolves as we grow older, but also enables us to distinguish normal healthy changes from pathological impairments. By identifying these changes earlier, we can take steps to maintain good brain health and mental sharpness as we get older. Ultimately, this can also lead to more efficient use of the resources in our healthcare system.
References
Bar, M. (2007). The proactive brain: using analogies and associations to generate predictions. Trends in Cognitive Sciences, 11(7), 280-289.
Burke, D. M., & Osborne, G. (2007). Aging and inhibition deficits: Where are the effects? In D. S. Gorfein & C. M. MacLeod (Eds.), Inhibition in cognition (pp. 163–183). American Psychological Association.
Cheimariou, S. (2016). Prediction in aging language processing. (PhD Dissertation), University of Iowa. Chemairou, 2016.
Clark, A. (2013). Whatever next? Predictive brains, situated agents, and the future of cognitive science. Behavioral and Brain Sciences, 36(03), 181-204.
DeLong, K. A., Urbach, T. P., & Kutas, M. (2005). Probabilistic word pre-activation during language comprehension inferred from electrical brain activity. Nature Neuroscience, 8(8), 1117-1121.
Dikker, S., Rabagliati, H., Farmer, T. A., & Pylkkänen, L. (2010). Early occipital sensitivity to syntactic category is based on form typicality. Psychological Science, 21(5), 629-634.
Engle, R. W. (2002). Working memory capacity as executive attention. Current Directions in Psychological Science, 11(1), 19–23.
Federmeier, K. D. (2007). Thinking ahead: The role and roots of prediction in language comprehension. Psychophysiology, 44(4), 491-505.
Ferreira, F., & Chantavarin, S. (2018). Integration and prediction in language processing: A synthesis of old and new. Current Directions in Psychological Science, 27(6),
Fjell, A. M., Sneve, M. H., Grydeland, H., Storsve, A. B., & Walhovd, K. B. (2016). The disconnected brain and executive function decline in aging. Cerebral Cortex, bhw082.
Harada, C. N., Love, M. C. N., & Triebel, K. L. (2013). Normal cognitive aging. Clinics in Geriatric Medicine, 29(4), 737-752.
Helmholtz, H. (1866/1962). Concerning the perceptions in general. In J. Southall (Ed.)
Treatise on physiological optics (3rd ed., Vol. III). New York: Dover, (Translation).
Huettig, F., & Janse, E. (2016). Individual differences in working memory and processing speed predict anticipatory spoken language processing in the visual world. Language, Cognition and Neuroscience, 31(1), 80-93.
Kukona, A., Cho, P. W., Magnuson, J. S., & Tabor, W. (2014). Lexical interference effects in sentence processing: Evidence from the visual world paradigm and selforganizing models. Journal of Experimental Psychology: Learning, Memory, & Cognition, 40(2), 326-347. doi: 10.1037/a0034903.
Kuperberg, G. R., & Jaeger, T. F. (2016). What do we mean by prediction in language comprehension? Language, Cognition and Neuroscience, 31(1), 32-59.
Lindenberger, U., & Baltes, P. B. (1994). Sensory functioning and intelligence in old age: a strong connection. Psychology and Aging, 9(3), 339-355. Lupyan, G., & Clark, A. (2015). Words and the world predictive coding and the language-perception-cognition interface. Current Directions in Psychological Science, 24(4), 279-284.
Mani, N., & Huettig, F. (2014). Word reading skill predicts anticipation of upcoming spoken language input: A study of children developing proficiency in reading. Journal of Experimental Child Psychology, 126, 264-279.
Pickering, M. J., & Garrod, S. (2013). An integrated theory of language production and comprehension. Behavioral and Brain Sciences, 36(04), 329-347.
Reifegerste, J., Hauer, F., & Felser, C. (2016). Agreement processing and attraction errors in aging: Evidence from subject-verb agreement in German. Aging Neuropsychology and Cognition, 24(6), 672-702.
Salthouse, T. A. (1996). The processing-speed theory of adult age differences in cognition. Psychological Review, 103(3), 403-428.
Stine-Morrow, E. A. L., Parisi, J. M., Morrow, D. G., Greene, J. C., & Park, D. C. (2007). An engagement model of cognitive optimization through Adulthood. The Journals of Gerontology: Series B, 62(Special_Issue_1), 62-69.